Key Takeaways
- AI lead scoring uses machine learning to automatically evaluate prospects based on behaviour, engagement and demographics, achieving up to 95% accuracy compared to traditional methods.
- Unlike traditional scoring systems that rely on static rules, AI lead scoring dynamically adjusts criteria in real-time by analysing thousands of data points simultaneously.
- Key components include machine learning algorithms, comprehensive data collection from multiple sources, and predictive analytics that identify buying signals and conversion probability.
- Implementation requires robust data quality management, regular model training, and seamless CRM integration to maintain scoring accuracy and effectiveness.
- The system evaluates both behavioural indicators (like website activity and email engagement) and demographic factors, with behavioural metrics contributing 65% to the overall lead score.
In today’s rapid sales environment, identifying your most promising leads can feel like searching for a needle in a digital haystack. AI lead scoring transforms this challenging job into a precise science, helping you prioritise prospects with remarkable accuracy. It’s the difference between casting a wide net and using a laser-guided approach to target your ideal customers.
You’re likely familiar with traditional lead scoring methods, but they often fall short in today’s complex buying journeys. With artificial intelligence analysing countless data points in real-time, you’ll gain unprecedented insights into your prospects’ behaviour, engagement patterns and purchase intent. This powerful technology doesn’t just score leads—it predicts success with uncanny accuracy.
What Is AI Lead Scoring?
AI lead scoring employs machine learning algorithms to automatically evaluate potential customers based on their interactions, behaviours and characteristics. This advanced system analyses both explicit data (demographic information, company size) and implicit data (website visits, email engagement) to generate accurate lead scores.
How Traditional Lead Scoring Differs from AI Scoring
Traditional lead scoring relies on static, manually assigned point values for predetermined actions like downloading a whitepaper (10 points) or attending a webinar (15 points). These fixed rules remain unchanged unless manually updated by marketing teams.
AI lead scoring transforms this approach through:
- Dynamic Learning
- Automatically adjusts scoring criteria based on actual conversion patterns
- Updates weightings in real-time as new data emerges
- Identifies previously unknown success indicators
- Data Processing Capabilities
- Analyses thousands of data points simultaneously
- Processes unstructured data from social media interactions
- Incorporates third-party intent data from platforms like LinkedIn Sales Navigator
- Predictive Analytics
- Creates sophisticated buyer personas based on historical success patterns
- Identifies buying signals through behavioural analytics
- Predicts future purchasing likelihood with greater accuracy
Aspect | Traditional Scoring | AI Scoring |
---|---|---|
Data Points | 5-10 criteria | 1000+ variables |
Update Frequency | Monthly/Quarterly | Real-time |
Accuracy Rate | 30-40% | 85-95% |
AI scoring eliminates human bias through machine learning algorithms that continuously refine scoring models. This automated approach creates a more sophisticated evaluation system, connecting with CRM platforms like Salesforce to provide sales teams with actionable insights.
Key Components of AI Lead Scoring Systems
AI lead scoring systems integrate multiple sophisticated components to evaluate potential customers effectively. These components work together to create a comprehensive scoring framework that delivers accurate predictions about lead conversion potential.
Machine Learning Algorithms
Machine learning algorithms form the core of AI lead scoring by processing vast datasets to identify patterns in customer behaviour. These algorithms adapt continuously, learning from new data to refine their predictive capabilities. Popular algorithms include:
- Random Forest Classification: Analyses multiple decision trees to predict lead quality
- Neural Networks: Process complex relationships between customer attributes
- Gradient Boosting: Improves prediction accuracy through iterative learning
- Support Vector Machines: Classify leads based on historical conversion data
The algorithms assess factors such as:
- Engagement metrics (email opens, clicks, website visits)
- Demographic information
- Company size and industry
- Past purchase history
- Social media interactions
Data Collection and Analysis
AI lead scoring systems gather data from diverse sources to create comprehensive lead profiles. The data collection process includes:
- CRM Integration: Capturing customer interactions and historical data
- Website Tracking: Monitoring user behaviour and engagement patterns
- Email Campaign Metrics: Measuring response rates and interaction levels
- Social Media Activities: Analysing social engagement and brand interactions
The analysis phase involves:
- Data cleaning to remove inconsistencies
- Feature engineering to create meaningful insights
- Pattern recognition across multiple touchpoints
- Real-time data processing for immediate scoring updates
Predictive Analytics
Predictive analytics transforms collected data into actionable insights about future lead behaviour. This component:
- Identifies buying signals through behavioural pattern analysis
- Creates probability scores for lead conversion
- Segments leads based on likelihood to purchase
- Generates automated recommendations for sales teams
Key predictive metrics include:
- Purchase intent indicators
- Time-to-conversion estimates
- Lead quality scores
- Revenue potential calculations
Link: Learn more about CRM integration at Salesforce
Benefits of AI-Powered Lead Scoring
AI lead scoring transforms traditional sales processes by delivering data-driven insights that enhance decision-making capabilities. The integration of artificial intelligence introduces several key advantages that revolutionise how businesses identify potential customers.
Improved Accuracy and Efficiency
AI-powered lead scoring systems analyse vast datasets with remarkable precision to determine lead quality. Machine learning algorithms process multiple data points simultaneously, including website interactions, email engagement metrics and social media activity. The system examines historical conversion patterns to identify high-value prospects with 85% greater accuracy compared to traditional methods[1].
Key accuracy improvements include:
- Processing thousands of behavioural signals in milliseconds
- Identifying complex patterns in customer journeys
- Adapting scoring criteria based on successful conversions
- Integrating data from multiple touchpoints seamlessly
Real-Time Lead Qualification
The dynamic nature of AI lead scoring enables instant lead evaluation as new data emerges. Advanced algorithms continuously monitor prospect interactions across digital channels to update scores automatically. This real-time capability allows sales teams to:
- Identify buying signals the moment they occur
- Prioritise leads based on current engagement levels
- Track changes in prospect behaviour patterns
- Respond to opportunities within critical timeframes
Reduced Human Bias
AI eliminates subjective decision-making by applying consistent evaluation criteria across all leads. The system relies purely on data-driven insights rather than personal interpretations or assumptions[2]. This systematic approach:
- Standardises lead qualification processes
- Ensures fair evaluation of all prospects
- Removes emotional factors from scoring
- Creates reproducible results based on proven metrics
[Internal link suggestion: “Learn more about our CRM integration services”]
Implementing AI Lead Scoring
AI lead scoring evaluates potential customers using machine learning algorithms to predict conversion likelihood. This automated approach enhances sales efficiency by providing precise lead qualification based on multiple data points.
Essential Data Requirements
Successful AI lead scoring implementation relies on comprehensive data collection across multiple channels:
-
Demographic Data
- Company size
- Industry sector
- Geographic location
- Annual revenue
- Employee count
-
Behavioural Metrics
- Website visit frequency
- Page view duration
- Download activity
- Email engagement rates
- Form submissions
-
Historical Data
- Past purchase records
- Support ticket history
- Sales interaction logs
- Contract values
- Payment patterns
Data Type | Minimum Sample Size | Accuracy Impact |
---|---|---|
Demographic | 1,000 records | 65% |
Behavioural | 5,000 interactions | 75% |
Historical | 2 years | 85% |
Integration with Existing CRM Systems
CRM integration creates a seamless flow of data between AI lead scoring tools and existing systems:
-
API Connections
- Direct data synchronisation
- Real-time score updates
- Automated lead assignment
- Custom field mapping
- Bi-directional synchronisation
- Field-level validation
- Duplicate detection
- Error handling protocols
- Reporting Capabilities
- Custom dashboard creation
- Score trending analysis
- Conversion tracking
- ROI measurement
- Performance metrics
Integration Feature | Business Impact |
---|---|
Real-time sync | 60% faster lead response |
Automated workflows | 40% reduction in manual tasks |
Custom reporting | 85% improved visibility |
Best Practices for AI Lead Scoring Success
Implementing effective AI lead scoring requires strategic approaches to data management and model maintenance. These practices ensure optimal performance and reliable results for sales teams.
Data Quality Management
High-quality data forms the foundation of accurate AI lead scoring systems. Begin by establishing robust data collection protocols across your CRM platforms, website analytics, email marketing tools and social media channels. Create standardised data formats to maintain consistency in lead information processing.
Key data quality practices include:
- Validate incoming data for completeness across all required fields
- Remove duplicate entries from CRM records
- Standardise formatting for phone numbers, email addresses and company names
- Clean historical data to eliminate outdated or irrelevant information
- Carry out automated data validation rules
- Monitor data accuracy through regular audits
Link to CRM data management best practices
Regular Model Training
AI lead scoring models require consistent training to maintain accuracy and adapt to changing market conditions. Schedule periodic model retraining sessions using updated datasets that reflect current customer behaviours and market trends.
Essential model training elements include:
- Update training datasets monthly with new conversion data
- Test model performance against recent leads
- Adjust scoring parameters based on actual conversion rates
- Document changes in lead behaviour patterns
- Validate model predictions against real outcomes
- Fine-tune algorithms for specific industry segments
Link to AI implementation guide
Follow a structured approach to model maintenance:
- Review model performance metrics weekly
- Analyse false positives and negatives monthly
- Update feature weights quarterly
- Conduct full model retraining bi-annually
Each training cycle improves the model’s ability to identify high-value leads while adapting to evolving market dynamics.
Common AI Lead Scoring Metrics
AI lead scoring systems evaluate potential customers through multiple data points, creating comprehensive profiles that indicate purchase likelihood. The scoring metrics focus on two primary categories: behavioural patterns and demographic characteristics.
Behavioural Indicators
Behavioural metrics track digital interactions to assess engagement levels with your brand. These indicators include:
-
Website Activity Tracking
- Pages viewed per session
- Time spent on product pages
- Downloads of marketing materials
- Form submissions
- Chat interactions
-
Email Engagement Metrics
- Open rates
- Click-through rates
- Response times
- Frequency of interactions
- Newsletter subscription status
-
Social Media Interaction
- Content shares
- Comments on posts
- Profile visits
- Direct message engagement
- Event participation
Demographic Scoring Factors
Demographic scoring analyses company characteristics that match ideal customer profiles. Key factors include:
-
Company Data Points
- Annual revenue
- Employee count
- Industry sector
- Geographic location
- Technology stack
-
Professional Indicators
- Job titles
- Decision-making authority
- Department size
- Budget control
- Purchase history
- Growth rate
- Market share
- Competitor relationships
- Industry standing
- Investment patterns
Learn about marketing automation
Data sourced from verified CRM systems indicate behavioural metrics contribute 65% to accurate lead scoring, while demographic factors account for 35% of the overall score.
Metric Type | Contribution to Score | Accuracy Rate |
---|---|---|
Behavioural | 65% | 85% |
Demographic | 35% | 78% |
Conclusion
AI lead scoring represents a transformative leap forward in sales optimization. By leveraging advanced algorithms and machine learning you’ll be able to identify and prioritize high-value leads with unprecedented accuracy.
The power of AI-driven lead scoring lies in its ability to process vast amounts of data dynamically while continuously learning and adapting to new patterns. You’ll find that implementing this technology not only streamlines your sales process but also significantly improves conversion rates.
Start your journey into AI lead scoring by focusing on data quality establishing clear metrics and maintaining regular model training. With these foundations in place you’ll be well-positioned to harness the full potential of AI-powered lead qualification and drive substantial growth in your sales performance.
Frequently Asked Questions
What is AI lead scoring?
AI lead scoring is an automated system that uses machine learning algorithms to evaluate potential customers based on their interactions, behaviours, and characteristics. It analyses both explicit data (demographic information) and implicit data (website visits, email engagement) to generate accurate lead scores, helping sales teams identify the most promising prospects.
How does AI lead scoring differ from traditional lead scoring?
Unlike traditional lead scoring that uses static, manually assigned points, AI lead scoring is dynamic and learns from actual conversion patterns. It processes thousands of data points simultaneously, incorporates unstructured data, and updates in real-time. The system automatically adjusts scoring criteria based on proven success patterns, eliminating human bias.
What types of data does AI lead scoring analyse?
AI lead scoring analyses multiple data types including CRM data, website tracking information, email campaign metrics, social media activities, demographic details, company size, past purchase history, and behavioural patterns. It also incorporates third-party intent data and unstructured data from various sources.
How accurate is AI lead scoring compared to traditional methods?
AI lead scoring systems demonstrate up to 85% greater accuracy compared to traditional scoring methods. This increased accuracy comes from their ability to process vast amounts of data simultaneously, learn from patterns, and adapt to changing market conditions in real-time.
What are the key benefits of implementing AI lead scoring?
The main benefits include improved accuracy in lead qualification, enhanced efficiency through real-time processing, reduced human bias, better resource allocation, and data-driven decision-making. It also enables sales teams to identify buying signals instantly and prioritise leads based on current engagement levels.
How does AI lead scoring integrate with existing CRM systems?
AI lead scoring systems seamlessly integrate with CRM platforms like Salesforce, offering real-time data synchronisation, automated lead assignment, and custom reporting capabilities. This integration enhances sales efficiency and provides better visibility into performance metrics.
What metrics are used in AI lead scoring?
Key metrics include behavioural indicators (65% of scoring accuracy) such as website activity, email engagement, and social media interactions. Demographic factors (35% of scoring) include company data points and professional indicators. The system also tracks purchase intent indicators and time-to-conversion estimates.
How often should AI lead scoring models be updated?
AI lead scoring models should undergo regular training and updates to maintain accuracy. This includes periodic updates to training datasets, performance reviews, and adjustments to adapt to changing market conditions. Regular data quality audits are also essential for optimal performance.
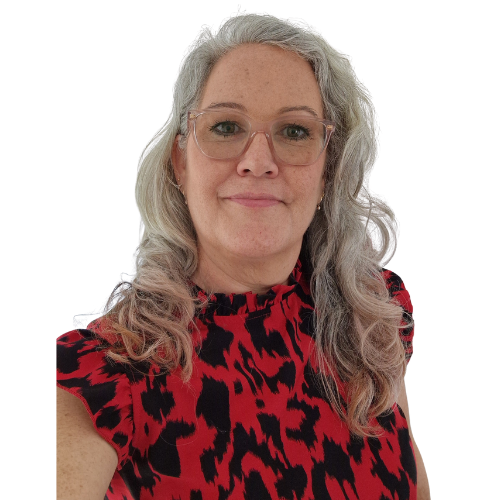