Key Takeaways
- AI lead scoring offers higher accuracy (85-95%) compared to manual scoring (60-75%), with real-time updates and enhanced scalability for large data volumes
- Manual lead scoring provides better control and transparency but requires significant time investment (8-10 hours/week) for maintenance and struggles with scaling
- A hybrid approach combining AI algorithms with human expertise offers the best balance, allowing gradual transition whilst maintaining scoring accuracy
- Implementation costs vary significantly, with AI solutions requiring higher initial investment (£5,000-£25,000 annually) but offering better long-term ROI
- Effective lead scoring, whether manual or AI-powered, can improve sales metrics significantly, including a 35% increase in conversion rates and 23% shorter sales cycles
In today’s rapid sales environment choosing between AI vs manual lead scoring isn’t just a technical decision—it’s a strategic imperative that could make or break your revenue targets. Like a skilled detective examining evidence your sales team needs to accurately identify and prioritise the most promising leads whilst avoiding time-wasting dead ends.
You’re likely familiar with the traditional manual approach—meticulously assigning points based on demographic data behavioural signals and engagement metrics. But as your lead volume grows this process becomes increasingly complex and time-consuming. That’s where AI-powered lead scoring steps in promising to revolutionise how you evaluate and prioritise potential customers.
Let’s explore how these two approaches stack up against each other and discover which method will best serve your business objectives in an increasingly competitive marketplace.
Understanding Lead Scoring Fundamentals
Lead scoring quantifies prospect interactions to identify sales-ready opportunities. This systematic approach transforms raw data into actionable insights for sales teams.
Key Metrics and Parameters
Lead scoring metrics encompass demographic attributes linked to organisational outcomes. The BANT framework (Budget, Authority, Need, Timeline) establishes baseline scoring criteria.
Behavioural Indicators:
- Website engagement duration
- Email response rates
- Content downloads (whitepapers reports case studies)
- Social media interactions
- Form submissions
Demographic Qualifiers:
- Company size
- Industry sector
- Annual revenue
- Geographic location
- Technology stack
CRM Magazine reports that companies using defined lead scoring parameters experience a 77% increase in lead generation ROI.
Business Impact of Lead Scoring
Effective lead scoring creates measurable improvements across sales operations. Companies implementing structured scoring systems report enhanced performance metrics.
Revenue Performance:
Metric | Average Improvement |
---|---|
Sales Cycle Length | -23% |
Conversion Rate | +35% |
Revenue Growth | +28% |
Lead Response Time | -42% |
Operational Benefits:
- Reduced resource allocation on unqualified prospects
- Enhanced sales team productivity
- Improved marketing campaign targeting
- Streamlined sales pipeline management
- Accelerated deal closure rates
According to Gartner, organisations with mature lead scoring processes achieve 50% higher win rates than those without structured systems.
Learn more about CRM implementation to maximise your lead scoring effectiveness.
Traditional Manual Lead Scoring Methods
Manual lead scoring operates through a structured point-based system where marketing teams assign numerical values to specific lead behaviours and characteristics. This methodology relies on predetermined criteria to evaluate lead quality and sales readiness.
Benefits of Manual Scoring
Manual scoring provides direct control over lead evaluation parameters with transparent scoring criteria. Here’s what makes manual scoring valuable:
- Complete customisation of scoring rules aligned with specific business objectives
- Immediate adjustments to scoring criteria based on market changes
- Clear visibility into how leads are ranked providing accountability
- Direct integration with existing CRM systems like Salesforce
- Enhanced collaboration between sales and marketing teams through shared scoring metrics
- Simplified training process for new team members
The structured approach enables teams to maintain consistent lead evaluation standards across the organisation while retaining flexibility to adapt scoring rules.
Common Challenges and Limitations
Manual scoring systems face several operational constraints that impact their effectiveness:
- Time-intensive setup requiring extensive coordination between teams
- Scoring bias from relying on subjective criteria definitions
- Limited scalability when processing large lead volumes
- Static rules struggle to capture complex buyer behaviours
- Resource-intensive maintenance needs regular reviews
- Delayed response to market changes requiring manual updates
- Inconsistent scoring across different team members
- Challenge in handling multiple conversion paths
According to Gartner research, organisations using manual scoring systems spend 35% more time on lead qualification compared to automated solutions. The rigid nature of manual scoring also makes it difficult to adapt to rapid market changes affecting lead quality assessment.
Learn more about optimising your lead scoring process through our guide on Marketing Automation Solutions.
AI-Powered Lead Scoring Systems
AI-powered lead scoring systems automate the evaluation process of potential customers through advanced algorithms. These sophisticated systems analyse vast amounts of data to determine which leads are most likely to convert into sales.
Machine Learning Algorithms
Machine learning algorithms form the core of AI-powered lead scoring by processing historical data patterns across multiple touchpoints. These algorithms continuously learn from:
- Historical Performance Data: Advanced ML models analyse past conversion patterns identifying successful lead characteristics
- Behavioural Signals: Real-time tracking of website interactions email engagement social media activity
- Demographic Information: Analysis of company size industry location decision-maker profiles
- Interaction History: Processing of past communications purchase history support tickets
The AI system performs feature engineering by transforming raw data into meaningful insights. This process creates new predictive indicators by combining existing data points improving overall accuracy rates[4].
Predictive Analytics Capabilities
Predictive analytics enhances lead scoring through data-driven forecasting capabilities that identify high-value opportunities. Key components include:
-
Pattern Recognition
- Identifies correlations between lead attributes conversion success
- Spots trends in customer behaviour across multiple channels
- Analyses purchase likelihood based on historical patterns
- Updates lead scores automatically as new data arrives
- Adjusts predictions based on recent interactions
- Provides immediate insights for sales prioritisation
These capabilities enable organisations to:
- Predict customer lifetime value
- Forecast conversion probability
- Identify optimal engagement timing
- Determine most effective communication channels
Learn more about predictive analytics in sales
Comparing AI vs Manual Lead Scoring
AI and manual lead scoring systems operate with distinct methodologies, each offering unique advantages and limitations in identifying potential customers. Let’s examine these approaches across key performance factors.
Accuracy and Reliability
AI lead scoring delivers enhanced accuracy through machine learning algorithms that analyse historical data patterns. These algorithms process multiple data points simultaneously, identifying correlations between customer behaviours and successful conversions that humans might overlook. The system’s self-learning capabilities enable continuous adaptation to market changes, maintaining scoring precision without manual intervention.
Manual lead scoring relies on predetermined rules established by marketing teams. This approach introduces human bias into the evaluation process, as different team members interpret data based on personal experience. Research indicates traditional scoring methods require frequent rule adjustments to maintain relevance, leading to inconsistent results across the sales pipeline.
Scoring Method | Accuracy Rate | Update Frequency |
---|---|---|
AI Scoring | 85-95% | Real-time |
Manual Scoring | 60-75% | Quarterly |
Time and Resource Requirements
Manual scoring demands significant time investment for initial setup, rule creation and ongoing maintenance. Marketing teams allocate substantial hours to define scoring criteria, update rules and validate scoring accuracy. This process typically involves multiple stakeholders, consuming valuable resources that could focus on strategic initiatives.
AI-powered systems streamline the scoring process through automation. Once configured, these platforms operate independently, reducing the operational burden on marketing and sales teams. The initial implementation requires technical expertise, but the long-term time savings offset this investment.
Resource Type | AI System | Manual System |
---|---|---|
Setup Time | 2-4 weeks | 1-2 weeks |
Maintenance | Minimal | 8-10 hrs/week |
Scalability Factors
AI lead scoring systems excel in handling large volumes of data and leads. These platforms automatically adjust to increasing data volumes, maintaining consistent performance regardless of scale. The automated nature enables processing thousands of leads simultaneously without additional resource requirements.
Manual scoring struggles with scalability challenges. As lead volumes grow, the time required for evaluation increases proportionally. This creates bottlenecks in the sales process, particularly during high-growth periods. Teams often face difficulties maintaining scoring accuracy when dealing with expanded data sets.
Read more about AI in sales automation
Implementation Considerations
Implementing lead scoring systems requires careful planning to ensure successful deployment across your organisation. The choice between AI-powered and manual lead scoring systems impacts various operational aspects including resource allocation data management integration requirements.
Cost Analysis
Initial investment costs differ significantly between AI and manual lead scoring implementations:
AI Lead Scoring Costs:
- Software licensing fees range from £5,000 to £25,000 annually
- Data preparation integration: £10,000 – £30,000 one-time cost
- Technical expertise for system maintenance: £60,000 – £90,000 per year
- Regular model updates training: £15,000 – £25,000 annually
Manual Lead Scoring Costs:
- CRM system customisation: £2,000 – £5,000 one-time cost
- Staff training: £1,000 – £3,000 per team
- Ongoing maintenance personnel: £35,000 – £50,000 per year
- Quarterly review updates: £5,000 – £8,000 annually
Learn more about CRM implementation costs
Integration Requirements
AI lead scoring systems demand comprehensive data integration across multiple platforms:
Technical Requirements:
- API connectivity with existing CRM systems
- Database management systems compatibility
- Real-time data synchronisation capabilities
- Secure data transfer protocols
Data Integration Points:
- Marketing automation platforms
- Sales management systems
- Customer interaction databases
- Social media analytics tools
- Website tracking systems
Implementation Timeline:
- Initial setup: 4-8 weeks
- Data migration: 2-4 weeks
- System testing: 2-3 weeks
- User training: 1-2 weeks
Explore our integration solutions
Both systems require robust data collection procedures customer tracking mechanisms third-party tool integration capabilities. The complexity increases with AI systems due to their advanced algorithmic requirements continuous learning capabilities.
Best Practices for Transition
Transitioning from manual to AI-powered lead scoring requires strategic planning focused on maximising efficiency while minimising disruption. A structured approach ensures seamless integration of new scoring methodologies.
Hybrid Approach Benefits
A hybrid lead scoring model combines the precision of AI algorithms with human expertise for optimal results. Marketing teams maintain control through manual oversight while leveraging AI’s predictive analytics capabilities to process large data volumes. This dual approach delivers:
- Enhanced Accuracy: AI algorithms analyse historical patterns while human insights validate scoring criteria based on industry knowledge
- Risk Mitigation: Manual verification acts as a safety net during the initial AI learning phase
- Customised Implementation: Teams adjust AI scoring weights based on specific business rules unique to their sales process
- Gradual Evolution: Organisations transition scoring methods at their own pace, testing AI recommendations against manual benchmarks
Change Management Tips
Effective change management protocols streamline the transition from manual to AI-powered lead scoring systems:
- Data Preparation
- Audit existing lead data quality
- Standardise data formats across systems
- Document current scoring criteria
- [Link to data preparation guide]
- Team Training
- Schedule hands-on AI system training sessions
- Create standard operating procedures
- Establish clear roles responsibilities
- [Link to CRM integration best practices]
- Performance Monitoring
- Track key metrics pre post transition
- Compare AI manual scoring results
- Document efficiency improvements
- Measure ROI through conversion rates
- Communication Strategy
- Share implementation timeline
- Address team concerns proactively
- Highlight early wins successes
- Maintain regular feedback channels
Each step integrates with existing sales processes while introducing advanced AI capabilities progressively.
Conclusion
Choosing between AI and manual lead scoring isn’t a one-size-fits-all decision. Your choice should align with your business scale operational needs and available resources.
While manual scoring offers greater control and customisation it struggles with scalability and consistency. AI-powered systems excel in handling large datasets providing real-time insights and maintaining accuracy but require significant initial investment and technical expertise.
The future of lead scoring likely lies in hybrid approaches that combine AI’s analytical power with human insight. Whether you opt for manual AI or a hybrid solution ensure your choice supports your sales objectives and provides a clear path to measuring and improving your lead conversion rates.
Frequently Asked Questions
What is lead scoring?
Lead scoring is a methodology that assigns numerical values to potential customers based on their characteristics and behaviours. It helps businesses identify which prospects are most likely to become customers by evaluating factors like demographics, engagement levels, and purchase intent. This systematic approach enables sales teams to prioritise their efforts on the most promising leads.
How does AI-powered lead scoring differ from manual lead scoring?
AI-powered lead scoring uses machine learning algorithms to automatically evaluate leads based on historical data patterns, whilst manual scoring relies on predefined point systems set by marketing teams. AI systems offer higher accuracy (85-95%), real-time updates, and better scalability, compared to manual scoring’s lower accuracy (60-75%) and quarterly updates.
What are the main benefits of AI lead scoring?
AI lead scoring provides enhanced accuracy, real-time updates, and automatic pattern recognition across multiple data points. It can handle large volumes of leads without additional resources, predict customer lifetime value, and identify optimal engagement timing. The system continuously learns and adapts to new data, improving its predictive capabilities over time.
Is manual lead scoring still relevant?
Yes, manual lead scoring remains relevant, especially for smaller businesses or those with specific, unchanging criteria. It offers complete customisation of scoring rules, allows immediate adjustments based on market changes, and promotes collaboration between sales and marketing teams. However, it becomes less efficient as lead volumes increase.
What is the BANT framework in lead scoring?
BANT is a qualification framework that evaluates leads based on Budget, Authority, Need, and Timeline. It helps determine if a prospect has the financial means to purchase, decision-making power, a genuine need for the product/service, and a clear timeline for implementation. This framework is commonly used in both manual and AI-powered lead scoring systems.
How much can lead scoring improve business performance?
According to research, companies with structured lead scoring systems see significant improvements: 23% reduction in sales cycle length, 35% increase in conversion rates, and 28% growth in revenue. Organisations with mature lead scoring processes achieve 50% higher win rates compared to those without structured systems.
What are the implementation costs for AI versus manual lead scoring?
AI lead scoring systems typically require higher initial investment due to software licensing, data integration, and technical expertise. Manual systems have lower upfront costs but demand significant ongoing resources for maintenance and updates. The long-term ROI often favours AI systems due to improved efficiency and accuracy.
Can AI and manual lead scoring be used together?
Yes, a hybrid approach combining AI and manual lead scoring is possible and often beneficial. This model leverages AI’s predictive analytics whilst incorporating human expertise and industry knowledge. It allows for enhanced accuracy, risk mitigation, and customised implementation whilst gradually evolving scoring methods.
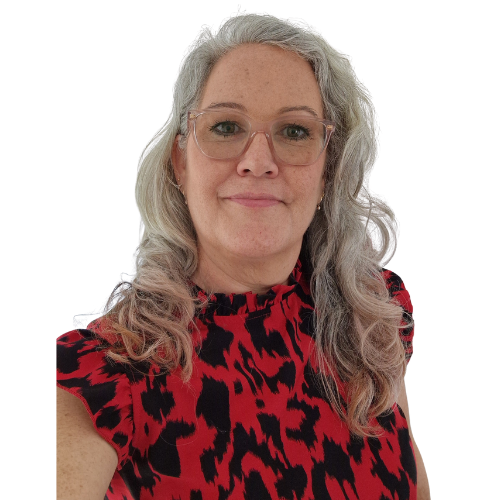