Key Takeaways
- AI lead scoring tools leverage machine learning algorithms to analyse multiple data points, helping sales teams identify and prioritise high-value prospects with up to 30% higher conversion rates
- Leading platforms like HubSpot, Salesforce Einstein, and Exceed.ai offer essential features including real-time scoring updates, CRM integration, and customisable scoring parameters
- Successful implementation relies on clean data management, proper system integration, comprehensive team training, and regular performance monitoring
- These tools deliver measurable ROI through reduced sales cycles (25% shorter), increased revenue per lead (35% growth), and improved operational efficiency
- Key features to look for include machine learning capabilities, robust integration options, and customisation features that adapt to your specific industry needs
In today’s rapid sales environment, finding the right AI lead scoring tools can feel like searching for a needle in a digital haystack. With countless options flooding the market, it’s crucial to identify solutions that truly deliver results rather than empty promises. Your sales team deserves better than manually sifting through endless leads, hoping to strike gold.
You’re likely familiar with the frustration of watching promising leads slip through the cracks while your team wastes precious time on prospects that never convert. Modern AI-powered lead scoring tools can transform this chaotic guesswork into a precise science, helping you identify and prioritise your most valuable prospects with remarkable accuracy. By leveraging advanced algorithms and machine learning, these sophisticated systems analyse countless data points to predict which leads are most likely to convert.
Understanding AI Lead Scoring: A Modern Sales Essential
AI lead scoring transforms raw customer data into actionable insights through sophisticated machine learning algorithms. These systems analyse multiple data points including website interactions, email engagement, social media activity [source: Gartner Research] to generate accurate lead values.
How AI Lead Scoring Works
Modern AI scoring platforms integrate with your existing CRM systems to process:
- Digital Footprints: Website navigation patterns, content downloads, form submissions
- Engagement Metrics: Email open rates, click-through rates, response times
- Demographic Data: Company size, industry sector, geographic location
- Behavioural Signals: Purchase history, service interactions, support tickets
The AI algorithms identify patterns in these datasets to calculate lead scores based on:
- Explicit Scoring: Direct information provided by leads
- Implicit Scoring: Behavioural data analysis
- Negative Scoring: Factors indicating reduced purchase likelihood
Benefits of AI-Powered Scoring
AI lead scoring delivers measurable improvements to sales processes:
Metric | Average Improvement |
---|---|
Lead Conversion Rate | 30% increase |
Sales Cycle Length | 25% reduction |
Revenue per Lead | 35% growth |
These systems excel at:
- Pattern Recognition: Identifying complex relationships in customer behaviour
- Real-time Updates: Continuously adjusting scores based on new interactions
- Predictive Analysis: Forecasting future lead behaviour based on historical data
- Scale Management: Processing thousands of leads simultaneously
Learn more about CRM integration with AI tools
AI lead scoring eliminates manual scoring inconsistencies through automated data analysis. The technology adapts to market changes by learning from new data patterns while maintaining scoring accuracy across your entire lead database.
Top AI Lead Scoring Platforms for 2025
AI-powered lead scoring platforms transform raw data into actionable insights through advanced algorithms and machine learning capabilities. These platforms analyse multiple data points to identify high-value prospects and prioritise sales efforts effectively.
HubSpot Lead Scoring AI
HubSpot’s AI lead scoring system combines engagement analysis with fit scoring to deliver comprehensive lead evaluation. The platform’s AI-Assisted Engagement Scoring examines successful lead interactions to identify promising prospects based on website visits and marketing campaign responses. The AI-Assisted Fit Scoring component evaluates leads against ideal customer profiles by analysing demographic factors like company size, industry type and revenue metrics.
Key features:
- Custom scoring parameters with time decay functionality
- Unified event tracking across marketing channels
- Advanced weighting algorithms for precise lead prioritisation
- Real-time engagement monitoring
- Seamless CRM integration
Salesforce Einstein Lead Scoring
Einstein Lead Scoring leverages predictive analytics to identify patterns in historical data and determine which leads are most likely to convert. The system processes extensive datasets including:
- Past conversion patterns
- Industry-specific metrics
- Behavioural signals
- Demographic information
- Engagement history
The platform automatically adjusts scoring models based on successful conversions, ensuring continuous optimisation of lead qualification processes.
Exceed.ai
Exceed.ai combines AI-driven lead scoring with automated engagement capabilities. The platform’s intelligent scoring system:
- Evaluates lead quality through natural language processing
- Automates personalised follow-up communications
- Tracks engagement levels across multiple touchpoints
- Identifies optimal engagement timing
- Integrates with existing marketing automation tools
The system’s machine learning algorithms adapt to specific business contexts, improving accuracy over time.
Leadfeeder
Leadfeeder employs website visitor tracking and AI scoring to identify high-potential leads. The platform’s capabilities include:
- Anonymous visitor identification
- Company profile enrichment
- Website behaviour analysis
- Custom scoring rules implementation
- CRM synchronisation
The system generates detailed visitor insights and automatically ranks leads based on engagement patterns and firmographic data.
Learn more about HubSpot’s AI capabilities
Key Features to Look for in AI Lead Scoring Tools
AI lead scoring tools transform raw data into valuable insights through sophisticated algorithms and automated analysis. These essential features determine the effectiveness of your lead scoring system.
Machine Learning Capabilities
Modern AI lead scoring platforms leverage advanced machine learning algorithms to analyse historical data and predict lead behaviour with remarkable accuracy. Salesforce Einstein exemplifies this capability by continuously refining its predictions based on new data and outcomes, improving lead qualification over time.
Key machine learning features include:
- Pattern recognition in lead engagement data
- Predictive analytics for conversion likelihood
- Automated score adjustments based on new interactions
- Behaviour analysis across multiple touchpoints
- Real-time learning from sales outcomes
HubSpot’s machine learning system tracks website interactions, email engagement and sales team communications to generate dynamic lead scores. This automated approach eliminates manual scoring inconsistencies and adapts to changing market conditions.
Integration Options
Effective AI lead scoring tools seamlessly connect with existing business systems:
- CRM platforms for centralised data management
- Marketing automation software
- Email marketing systems
- Website analytics tools
- Sales engagement platforms
Marketo Engage demonstrates strong integration capabilities by:
- Syncing lead data across multiple platforms
- Providing real-time score updates to CRM systems
- Enabling automated workflow triggers
- Consolidating engagement metrics from various sources
- Supporting bi-directional data flow
Customisation Features
Advanced customisation options allow organisations to tailor scoring models:
- Custom scoring parameters based on industry
- Weighted scoring criteria for different actions
- Adjustable threshold levels for lead qualification
- Industry-specific engagement metrics
- Personalised scoring rules for different products
Leading platforms offer flexible configuration options:
- Rule-based scoring adjustments
- Custom field mapping
- Segmentation criteria modification
- Automated workflow customisation
- Scoring model templates for different industries
Link to HubSpot’s AI Lead Scoring Features
Measuring ROI from AI Lead Scoring Solutions
Quantifiable Performance Metrics
AI lead scoring tools generate measurable improvements in sales performance through data-driven insights. Leading platforms track key performance indicators including conversion rates, sales cycle duration and revenue per lead. Implementation success metrics reveal:
Metric | Improvement |
---|---|
Lead Conversion Rate | 30% increase |
Sales Cycle Length | 25% reduction |
Revenue per Lead | 35% growth |
Integration Benefits
CRM integration amplifies ROI potential by streamlining data flow between systems. Salesforce Einstein and HubSpot Lead Scoring connect seamlessly with existing platforms, eliminating manual data entry and improving efficiency. The automated lead qualification process reduces resource requirements while increasing accuracy.
Productivity Enhancements
AI-powered scoring tools transform sales productivity through:
- Automated lead prioritisation based on engagement patterns
- Real-time scoring updates reflecting prospect interactions
- Intelligent routing of qualified leads to appropriate team members
- Dynamic adjustment of scoring models based on conversion data
- Elimination of manual scoring inconsistencies
Cost Reduction Analysis
Implementation of AI lead scoring reduces operational costs by:
- Decreasing time spent on unqualified leads
- Minimising manual data entry requirements
- Optimising sales resource allocation
- Reducing customer acquisition costs
- Automating lead nurturing processes
The predictive analytics capabilities enable sales teams to focus efforts on high-value prospects, maximising resource utilisation and improving conversion rates. This targeted approach delivers substantial returns through increased sales efficiency and reduced operational costs.
Implementation Best Practices for AI Lead Scoring
AI lead scoring implementation requires strategic planning and precise execution to maximise effectiveness. Here are the essential practices for optimal implementation:
Data Quality Management
- Clean your existing customer data before migration
- Standardise data input formats across all channels
- Establish regular data validation protocols
- Create automated data enrichment processes
- Monitor data accuracy through periodic audits
Scoring Model Configuration
- Define clear scoring criteria based on your ideal customer profile
- Assign weighted values to different behavioural signals
- Create separate scoring models for different product lines
- Carry out negative scoring for disqualifying actions
- Set up regular model performance reviews every 90 days
Integration Strategy
- Sync your CRM system with Salesforce Einstein for seamless data flow
- Connect marketing automation platforms to track engagement metrics
- Integrate email marketing tools for comprehensive lead tracking
- Establish bi-directional data synchronisation
- Set up real-time alert systems for high-scoring leads
Team Training
- Train sales teams on interpreting AI-generated scores
- Create standardised processes for lead follow-up
- Develop response protocols for different score thresholds
- Establish clear handoff procedures between teams
- Document best practices for ongoing reference
Performance Monitoring
- Track conversion rates by score range
- Measure average deal size against lead scores
- Monitor sales cycle length variations
- Calculate ROI through HubSpot’s analytics tools
- Analyse false positive rates to refine scoring models
Each practice strengthens your AI lead scoring implementation, creating a robust system that accurately identifies high-value prospects while maintaining efficiency in lead management processes.
Conclusion
AI lead scoring tools have revolutionised how businesses identify and nurture potential customers. By leveraging advanced algorithms machine learning and real-time analytics these platforms transform raw data into actionable insights that drive measurable results.
The right AI lead scoring solution will seamlessly integrate with your existing systems while offering customisation options that align with your unique business needs. Whether you choose HubSpot Salesforce Einstein or another leading platform you’ll benefit from enhanced conversion rates shorter sales cycles and improved revenue per lead.
Remember, successful implementation depends on clean data proper configuration and regular performance monitoring. With the right approach AI lead scoring becomes an invaluable asset in your sales toolkit helping you focus on the leads that matter most.
Frequently Asked Questions
What is AI lead scoring?
AI lead scoring is an automated system that uses artificial intelligence and machine learning to evaluate and rank potential customers based on their likelihood to convert. It analyses various data points like website interactions, email engagement, and social media activity to assign scores to leads, helping sales teams prioritise their efforts more effectively.
How does AI lead scoring improve sales performance?
AI lead scoring has demonstrated significant improvements in sales performance, including a 30% increase in lead conversion rates, 25% reduction in sales cycle length, and 35% growth in revenue per lead. It achieves this by accurately identifying high-value prospects and eliminating time wasted on unqualified leads.
Which are the top AI lead scoring platforms in 2025?
The leading AI lead scoring platforms include HubSpot, Salesforce Einstein, Exceed.ai, and Leadfeeder. Each platform offers unique features: HubSpot combines engagement analysis with fit scoring, Salesforce Einstein uses predictive analytics, Exceed.ai integrates scoring with automated engagement, and Leadfeeder specialises in website visitor tracking.
What features should I look for in an AI lead scoring tool?
Key features to consider include advanced machine learning capabilities, seamless integration options with existing CRM systems, customisation features, real-time learning capabilities, and automated score adjustments. The tool should also offer detailed analytics and reporting functionalities.
How do I measure the ROI of AI lead scoring?
Track key performance metrics such as lead conversion rates, sales cycle length, and revenue per lead. Also monitor operational cost reductions, time saved on lead qualification, and improvements in sales resource allocation. Regular analysis of these metrics helps demonstrate the solution’s value and identify areas for optimisation.
What are the best practices for implementing AI lead scoring?
Focus on maintaining high-quality data through regular cleaning and validation. Configure scoring models with clear criteria and weighted values, ensure seamless integration with existing systems, provide comprehensive team training, and consistently monitor performance metrics to refine the scoring model over time.
Can AI lead scoring integrate with existing CRM systems?
Yes, modern AI lead scoring tools are designed to integrate seamlessly with popular CRM platforms. This integration enables automatic data flow, enhances efficiency, and allows for real-time updates to lead scores based on new information and interactions.
How often should lead scoring models be updated?
Lead scoring models should be reviewed and updated regularly, typically quarterly, to ensure they remain accurate and relevant. Market changes, new product launches, or shifts in customer behaviour patterns may necessitate more frequent adjustments to maintain optimal performance.
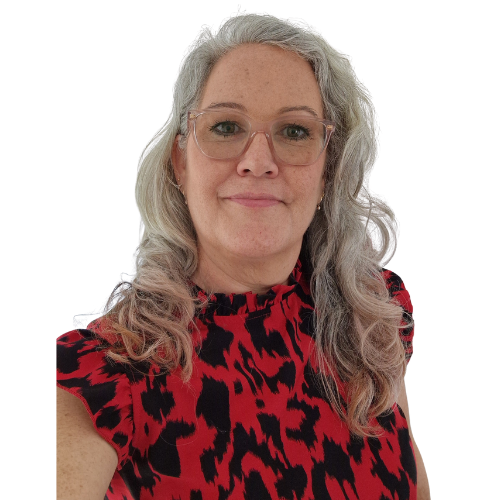