Key Takeaways
- AI transforms lead qualification by analysing multiple data points to identify prospects with genuine buying intent, improving sales efficiency by 30-40%
- Machine learning algorithms track digital footprints across website interactions, email responses, content downloads and social media activity to build comprehensive behaviour profiles
- Predictive lead scoring models combine engagement metrics, behavioural patterns and firmographic data to create precise qualification profiles with real-time updates
- Automated lead nurturing workflows deliver personalised content and follow-up sequences based on prospect behaviour, reducing sales cycles by 20-25%
- Implementation success requires maintaining high data quality standards (85%+ completeness) and seamless CRM integration with real-time synchronisation
Tired of chasing cold leads that go nowhere? AI identifies sales-ready leads with remarkable precision, transforming your sales pipeline from a guessing game into a strategic masterpiece. Like a skilled detective, artificial intelligence analyses countless data points to spot the subtle signals that indicate genuine buying intent.
Picture your sales team spending their valuable time engaging with prospects who are genuinely interested and ready to buy. Instead of drowning in a sea of unqualified leads, AI empowers you to focus on opportunities with the highest conversion potential. You’ll finally break free from the exhausting cycle of pursuing leads that aren’t worth your time.
Ready to revolutionise your sales approach? Learn how AI-powered lead qualification can help you achieve higher conversion rates, shorter sales cycles and increased revenue. Don’t let valuable opportunities slip through your fingers – discover how intelligent automation can transform your lead generation strategy.
Understanding AI-Powered Lead Qualification
AI-powered lead qualification systems process massive datasets to identify prospects most likely to convert. These advanced algorithms continuously learn from interactions to refine their predictive accuracy in determining sales readiness.
How Machine Learning Analyses Lead Behaviour
Machine learning algorithms track digital footprints across multiple touchpoints to evaluate prospect engagement patterns. The systems examine website interactions, email responses, content downloads and social media activity to build comprehensive behaviour profiles.
A crucial component of AI lead scoring involves natural language processing analysis of:
- Email conversations examining response times sentiment mood
- Chat interactions revealing specific pain points product interests
- Social media engagement indicating brand affinity purchase intent
- Form submissions highlighting budget authority timeline
The AI models automatically adjust scoring weights based on historical conversion data. For example, prospects downloading pricing guides receive higher scores than those viewing general blog content. This dynamic scoring ensures lead qualification accuracy improves over time through continuous learning.
Key Data Points That Signal Sales Readiness
AI systems evaluate specific data signals to determine prospect qualification status:
Data Point Category | Key Indicators | Impact Score |
---|---|---|
Engagement Metrics | Time on site, Pages viewed, Return visits | 35% |
Content Interaction | Downloads, Video views, Tool usage | 25% |
Company Information | Revenue, Employee count, Industry | 20% |
Behavioural Signals | Search patterns, Click paths, Form fills | 20% |
Critical qualification signals include:
- Multiple decision-maker interactions from same organisation
- Engagement with bottom-funnel content like pricing case studies
- Repeated visits to product comparison implementation pages
- Direct inquiries about integration technical specifications
- Budget-related searches document downloads
The AI correlates these signals with firmographic data creating comprehensive qualification profiles. This multi-dimensional analysis enables precise identification of high-value opportunities requiring immediate sales attention.
Predictive Lead Scoring Models
Predictive lead scoring models leverage AI algorithms to analyse multiple data points for identifying sales-ready leads. These sophisticated systems transform raw data into actionable insights by combining behavioural patterns demographic information CRM data.
Engagement Metrics and Scoring Parameters
AI-powered predictive models track specific engagement indicators across digital touchpoints:
- Website Interactions: Page visits content downloads form submissions session duration
- Email Engagement: Open rates click-through rates response times frequency of interactions
- Content Consumption: Whitepaper downloads case study views webinar attendance product documentation access
- Social Media Activity: Platform engagement post interactions professional network connections
The scoring parameters integrate with third-party data sources creating a comprehensive evaluation matrix:
Scoring Parameter | Weight | Impact Level |
---|---|---|
Website Visits | 30% | High |
Email Response | 25% | High |
Content Downloads | 20% | Medium |
Social Engagement | 15% | Medium |
Third-party Data | 10% | Low |
Historical Data Training Process
The model’s training process incorporates historical conversion data to enhance prediction accuracy:
- Data Collection Phase
- Gathering past customer journey information
- Documenting successful conversion patterns
- Recording deal closure timelines
- Mapping decision-maker interactions
- Pattern Recognition
- Identifying common characteristics of converted leads
- Analysing successful deal velocities
- Evaluating engagement sequences
- Measuring response intervals
- Model Refinement
- Continuous algorithm adjustments based on new data
- Performance metric tracking
- Accuracy rate monitoring
- Predictive pattern updates
Learn more about AI-powered lead scoring
Real-Time Lead Classification Systems
AI-powered classification systems analyse lead data instantaneously, enabling precise categorisation of prospects based on their likelihood to convert. These systems process multiple data points simultaneously to deliver accurate lead scoring in real-time.
Automated Lead Nurturing Workflows
AI-driven nurturing workflows transform lead engagement through sophisticated automation. The system tracks digital interactions across multiple touchpoints, automatically adjusting communication sequences based on prospect behaviour. Each interaction triggers personalised responses, from targeted email campaigns to customised content recommendations.
Key automation capabilities include:
- Dynamic content delivery based on prospect engagement levels
- Automated follow-up sequences triggered by specific actions
- Custom nurturing paths aligned with buyer journey stages
- Multi-channel engagement orchestration
- Real-time response mechanisms
These workflows integrate with CRM systems to maintain detailed interaction records while continuously optimising engagement strategies based on performance data.
Priority-Based Lead Distribution
Priority-based distribution mechanisms leverage AI algorithms to route leads to the most suitable sales representatives. The system analyses firmographic data coupled with behavioural signals to determine lead priority levels and optimal assignment patterns.
Distribution criteria encompass:
- Lead score thresholds based on engagement metrics
- Industry-specific expertise matching
- Territory alignment requirements
- Sales representative capacity balancing
- Response time optimisation
Predictive analytics power these distribution decisions by:
- Calculating lead conversion probability
- Identifying optimal engagement timing
- Matching lead characteristics with sales expertise
- Evaluating historical performance patterns
- Monitoring real-time availability
The system automatically adjusts distribution rules based on performance feedback loops, ensuring continuous improvement in lead assignment accuracy. Each successful conversion reinforces the AI model’s understanding of effective matching patterns.
Benefits of AI Lead Identification
AI-powered lead identification transforms sales processes by automating prospect evaluation and prioritisation. Advanced algorithms analyse multiple data points to identify high-value opportunities with remarkable precision.
Improved Sales Team Efficiency
AI automation revolutionises sales team productivity by eliminating manual lead qualification tasks. Sales representatives save 5-7 hours weekly through automated data entry, lead nurturing sequences and follow-up communications. The system processes real-time engagement data across multiple channels, including:
- Website interactions
- Email responses
- Content downloads
- Social media engagement
- Form submissions
This automated analysis enables sales teams to focus exclusively on pursuing qualified prospects rather than wasting time on unproductive leads. Integration with CRM systems provides seamless access to enriched prospect data, empowering representatives to have more meaningful conversations.
Higher Conversion Rates
AI lead identification directly impacts conversion metrics by precisely targeting prospects demonstrating genuine buying intent. Companies implementing AI-powered lead scoring experience:
Metric | Improvement |
---|---|
Lead conversion rate | 30-40% increase |
Sales cycle length | 20-25% reduction |
Revenue per lead | 35% growth |
The system continuously refines its predictive accuracy by:
- Analysing historical conversion patterns
- Tracking engagement across multiple touchpoints
- Evaluating prospect behaviour signals
- Correlating firmographic data
- Adjusting scoring weights dynamically
Learn more about AI-powered sales automation
This data-driven approach ensures sales efforts concentrate on prospects most likely to convert, maximising resource utilisation and revenue generation potential.
Implementation Best Practices
AI-powered lead identification demands specific implementation strategies to maximise effectiveness. The integration process requires careful attention to data quality standards paired with seamless CRM system compatibility.
Data Quality Requirements
Quality data forms the foundation of accurate AI-driven lead identification. Clean consistent data enables AI algorithms to identify patterns effectively generating reliable lead scores.
Key data quality requirements include:
- Data Completeness: Capturing essential firmographic data company size revenue industry location
- Data Accuracy: Maintaining up-to-date contact information validated email addresses verified phone numbers
- Data Standardisation: Implementing uniform formatting for fields dates currencies company names
- Real-time Updates: Syncing engagement data from multiple touchpoints website visits email interactions social media activity
- Data Enrichment: Incorporating third-party data sources to supplement existing information
Data Quality Metric | Minimum Requirement |
---|---|
Data Completeness | 85% field completion |
Data Accuracy | 95% validity rate |
Update Frequency | Real-time/hourly sync |
Integration With Existing CRM Systems
CRM integration ensures seamless data flow between AI tools sales platforms. The integration process connects AI algorithms with existing customer data creating a unified view of each lead.
Essential integration components include:
- API Connections: Establishing secure data transfer protocols between systems
- Field Mapping: Aligning data fields across platforms for consistent information flow
- Automated Synchronisation: Creating bi-directional data sync between AI tools CRM platforms
- Custom Workflows: Developing automated processes for lead scoring assignment routing
- Performance Tracking: Implementing analytics to measure integration effectiveness
Integration Metric | Target Performance |
---|---|
Data Sync Speed | < 5 minute delay |
System Uptime | 99.9% availability |
API Response Time | < 2 seconds |
Conclusion
AI-powered lead identification represents a game-changing advancement in modern sales processes. By leveraging sophisticated algorithms and machine learning capabilities you’ll transform how your team identifies and pursues sales opportunities.
The combination of predictive analytics real-time tracking and automated workflows ensures that your sales representatives focus their energy on leads most likely to convert. This targeted approach not only boosts conversion rates but also significantly reduces time spent on unqualified prospects.
As AI technology continues to evolve you’ll find even more sophisticated ways to identify and nurture sales-ready leads. Now’s the time to embrace this powerful tool and gain a competitive edge in your industry.
Frequently Asked Questions
What is AI-powered lead qualification?
AI-powered lead qualification uses artificial intelligence to analyse vast amounts of data and identify potential customers most likely to make a purchase. The system processes information from various touchpoints, including website visits, email interactions, and social media engagement, to determine a prospect’s buying intent and readiness to convert.
How does AI improve the sales process?
AI enhances sales efficiency by automating prospect evaluation and prioritisation, saving sales teams 5-7 hours weekly on manual tasks. It increases lead conversion rates by 30-40%, reduces sales cycle length by 20-25%, and grows revenue per lead by 35% by helping teams focus on the most promising opportunities.
What data does AI analyse for lead scoring?
AI analyses multiple data points including engagement metrics, content interaction, company information, and behavioural signals. It also processes email conversations, chat interactions, social media engagement, and form submissions. This information is combined with firmographic data to create comprehensive qualification profiles.
How accurate is AI-powered lead scoring?
AI lead scoring systems continuously learn and improve their accuracy through machine learning algorithms. The systems analyse historical conversion data and adjust scoring weights dynamically. As more data is processed and patterns are recognised, the predictive accuracy of the system increases over time.
What are the key requirements for implementing AI lead identification?
Successful implementation requires high-quality data that is complete, accurate, and standardised. The system must integrate seamlessly with existing CRM systems through API connections, with automated data synchronisation and custom workflows. Regular updates and system reliability are essential for maximum effectiveness.
How does AI prioritise lead distribution?
AI algorithms analyse firmographic data and behavioural signals to determine lead priority levels and match leads with the most suitable sales representatives. The system uses predictive analytics and performance feedback loops to continuously improve lead assignment accuracy and optimise distribution patterns.
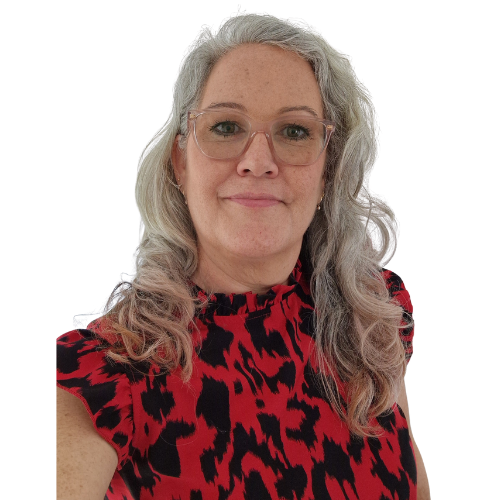