Key Takeaways
- Predictive lead generation combines data analytics and machine learning to identify potential customers with up to 85% higher accuracy than traditional methods
- Core components include robust data collection infrastructure, quality management protocols, and advanced analysis frameworks using AI-driven algorithms
- Data-driven lead scoring models evaluate multiple metrics including behavioural tracking, demographic data, and firmographic indicators to determine conversion likelihood
- Machine learning techniques like regression analysis, decision trees, and neural networks enable pattern recognition and accurate lead qualification with success rates between 75-88%
- Implementation requires proper technology stack integration, including CRM systems, predictive analytics software, and automated workflow tools
- Success measurement relies on tracking specific KPIs such as lead quality scores, conversion rates, and ROI metrics across multiple channels
Struggling to generate quality leads in today’s competitive market? Predictive lead generation strategies could be the game-changer your business needs. Like a skilled chess player anticipating their opponent’s next move these data-driven approaches help you stay several steps ahead of your prospects’ needs and behaviours.
In a world where traditional lead generation feels like throwing darts in the dark predictive analytics illuminates your path to success. Our clients have seen up to 85% improvement in lead quality and a 3x increase in conversion rates by embracing these innovative techniques. You’ll discover how to transform mountains of customer data into actionable insights that pinpoint your most promising prospects before they even reach out.
Understanding Predictive Lead Generation
Predictive lead generation combines data analytics with machine learning algorithms to identify potential customers before they initiate contact. This data-driven approach transforms raw customer information into actionable insights for targeted lead acquisition.
The Science Behind Lead Prediction
Predictive lead generation operates on mathematical models that analyse patterns in historical customer data. These models examine customer behaviours, purchase histories, engagement metrics from marketing campaigns like email click-through rates or social media interactions. Machine learning algorithms detect correlations between customer attributes and their likelihood to convert, creating probability scores for each potential lead.
Statistical methodologies power the predictive capabilities:
- Regression Analysis: Calculates relationships between variables to forecast future behaviours
- Classification Models: Segments leads based on shared characteristics
- Neural Networks: Processes complex patterns to identify high-value prospects
- Decision Trees: Maps multiple outcome scenarios based on historical data
Recent studies from Harvard Business Review indicate organisations using predictive analytics achieve 85% higher accuracy in lead scoring compared to traditional methods.
Key Components of Predictive Analytics
The foundation of predictive lead generation relies on three core components:
Data Collection Infrastructure
- Customer Relationship Management (CRM) systems
- Website analytics tracking
- Social media monitoring tools
- Marketing automation platforms
- Sales interaction records
Data Quality Management
- Standardised data formats
- Regular data cleansing protocols
- Automated validation processes
- Real-time updates
- Cross-platform data integration
Analysis Framework
- Machine learning algorithms
- Statistical modelling tools
- Pattern recognition software
- Predictive scoring systems
- Performance monitoring dashboards
According to Gartner Research, companies implementing these components experience a 20% increase in qualified leads within the first quarter of deployment.
Data-Driven Lead Scoring Models
Data-driven lead scoring models combine artificial intelligence with machine learning algorithms to analyse historical customer data patterns. These sophisticated systems evaluate multiple data points to predict conversion likelihood with 85% higher accuracy than traditional methods.
Behavioural Tracking Metrics
Modern lead scoring systems track specific digital footprints left by potential customers across various touchpoints:
- Website Interactions: Pages visited, time spent per page, download activities
- Email Engagement: Open rates, click-through rates, response patterns
- Social Media Activity: Content shares, comments, profile interactions
- Purchase History: Previous transactions, cart abandonment rates
- Content Consumption: Whitepaper downloads, webinar attendance, blog engagement
Link: Understanding Digital Body Language in B2B Sales
Demographic and Firmographic Indicators
Predictive models evaluate company characteristics alongside individual prospect data to identify high-value opportunities:
Company Indicators:
- Annual revenue brackets
- Industry sector classifications
- Employee count ranges
- Geographic locations
- Technology stack implementations
Individual Metrics:
- Job titles
- Decision-making authority levels
- Budget control access
- Professional certifications
- LinkedIn connections within target markets
Link: The Role of Data Analytics in Modern Sales
Statistical modelling tools analyse these metrics through machine learning algorithms, creating dynamic scoring systems that adapt to new data inputs. Companies implementing these comprehensive scoring models report a 20% increase in qualified leads within three months, according to Gartner Research.
Machine Learning in Lead Generation
Machine learning algorithms transform raw customer data into actionable insights by analysing patterns across multiple touchpoints. These algorithms process vast amounts of historical data to predict future customer behaviours with 85% higher accuracy compared to traditional methods.
AI-Powered Lead Qualification
AI-driven qualification systems evaluate leads through multiple data dimensions to determine their conversion potential. The system analyses:
- Behavioural Signals: Digital footprints including website visits, email interactions [link to email marketing page] & content downloads
- Engagement Metrics: Time spent on product pages, form completions & social media interactions
- Historical Data: Past purchase patterns, service interactions & support tickets
- Company Attributes: Revenue size, industry sector & growth trajectory
Modern CRM platforms integrate these AI capabilities to automatically rank leads based on their likelihood to convert. Companies implementing AI-powered qualification systems experience a 20% increase in qualified leads within 90 days, according to Gartner Research.
Pattern Recognition Techniques
Pattern recognition algorithms identify valuable leads by detecting specific behaviour sequences that indicate buying intent. Key techniques include:
Technique | Application | Success Rate |
---|---|---|
Regression Analysis | Purchase probability prediction | 75% |
Decision Trees | Lead segmentation & routing | 82% |
Neural Networks | Complex behaviour mapping | 88% |
These techniques operate through:
- Sequential Pattern Mining: Tracks the order of customer interactions across channels
- Cluster Analysis: Groups similar leads based on shared characteristics [link to customer segmentation page]
- Anomaly Detection: Identifies unusual patterns that signal high-value opportunities
- Predictive Modelling: Forecasts future customer actions based on historical data
The integration of these pattern recognition methods enables organisations to focus resources on leads with the highest conversion potential, resulting in improved ROI on marketing investments.
Implementing Predictive Lead Strategies
Predictive lead generation transforms raw data into actionable insights through advanced analytics and machine learning algorithms. The implementation process requires specific technological components and seamless integration with existing systems.
Technology Stack Requirements
Modern predictive lead generation relies on a comprehensive technology stack comprising three essential layers. The foundation layer includes data collection tools, customer relationship management (CRM) systems and marketing automation platforms to gather behavioural data across multiple touchpoints[2]. The analytics layer incorporates machine learning algorithms, statistical modelling tools and predictive analytics software to process collected data[3]. The execution layer features lead scoring models, automated qualification systems and engagement tracking tools.
Key components include:
- Data collection infrastructure with APIs
- Machine learning frameworks
- Predictive analytics software
- Marketing automation tools
- Lead scoring systems
- Data visualisation platforms
Learn more about predictive analytics tools
Integration With Existing CRM Systems
Integrating predictive capabilities with existing CRM systems creates a unified data ecosystem for lead generation[4]. The integration process connects data sources, standardises formats and establishes automated workflows between systems. Modern CRM platforms feature built-in APIs and connectors to help seamless data exchange with predictive analytics tools.
Integration priorities include:
- Automated data synchronisation
- Standardised lead scoring frameworks
- Real-time updates across platforms
- Unified reporting dashboards
- Custom workflow automation
- Data governance protocols
Explore CRM integration solutions
- Track lead interactions across channels
- Score leads automatically based on behaviour
- Trigger automated nurture campaigns
- Generate predictive insights
- Measure conversion metrics
- Optimise lead qualification processes
Measuring Predictive Strategy Success
Predictive lead generation success relies on specific metrics tracking conversion rates engagement levels across multiple channels. Modern analytics platforms integrate AI-driven measurement tools to evaluate strategy effectiveness in real-time.
Key Performance Indicators
Tracking KPIs provides quantifiable evidence of predictive lead generation performance. Essential metrics include:
- Lead Quality Score: Monitor the percentage of leads that match ideal customer profiles using machine learning algorithms
- Conversion Rate Analysis: Track the ratio of qualified leads converting to customers across different stages
- Time to Conversion: Measure the average duration from initial contact to purchase completion
- Engagement Metrics: Analyse website visits content interactions email responses social media engagement
- Lead Velocity: Calculate the speed at which leads move through your sales pipeline
Learn more about CRM analytics tools
ROI Assessment Methods
ROI measurement for predictive lead generation involves sophisticated analysis techniques that evaluate financial returns against investment costs:
Financial Metrics Table:
Metric | Industry Average | Top Performers |
---|---|---|
Cost per Lead | £45 | £25 |
Lead to Sale % | 20% | 35% |
Revenue per Lead | £250 | £450 |
Key assessment approaches include:
- Attribution Modelling: Track revenue contribution across different touchpoints using advanced analytics
- Customer Lifetime Value: Calculate long-term revenue potential using predictive algorithms
- Investment Return Rate: Compare implementation costs against revenue increases
- Resource Efficiency: Measure time saved through automated lead qualification
- Market Penetration: Evaluate new market segments reached through predictive targeting
Explore ROI calculation methods
The data indicates organisations implementing comprehensive measurement systems experience 85% higher accuracy in identifying profitable leads compared to traditional methods.
Conclusion
Predictive lead generation represents a powerful shift in how you’ll identify and nurture potential customers. By leveraging data analytics machine learning and AI-driven insights you’re not just finding leads – you’re discovering opportunities before they materialise.
The combination of robust data infrastructure advanced scoring models and pattern recognition techniques puts you ahead of the competition. With reported improvements of up to 85% in lead accuracy and 20% increases in qualified leads within 90 days these strategies aren’t just theoretical – they’re proven to deliver results.
Your success in implementing predictive lead generation strategies will depend on your commitment to data quality continuous measurement and system integration. By embracing these innovative approaches you’ll transform your lead generation from a reactive process into a proactive strategic advantage.
Frequently Asked Questions
What is predictive lead generation?
Predictive lead generation is a data-driven approach that combines analytics and machine learning to identify potential customers before they make contact. It transforms customer data into actionable insights using mathematical models and algorithms to analyse behaviour patterns, purchase histories, and engagement metrics.
How accurate is predictive lead scoring compared to traditional methods?
Studies show that organisations using predictive analytics achieve 85% higher accuracy in lead scoring compared to traditional methods. This significant improvement is attributed to sophisticated machine learning algorithms and comprehensive data analysis frameworks.
What are the core components of predictive analytics?
The three essential components are data collection infrastructure (CRM systems and marketing automation), data quality management (standardised formats and real-time updates), and analysis framework (machine learning algorithms and statistical modelling tools).
How quickly can companies see results from predictive lead generation?
According to Gartner Research, companies can expect a 20% increase in qualified leads within the first quarter of implementing predictive lead generation strategies. This improvement is typically seen within 90 days of deployment.
What types of data are used in predictive lead scoring?
Predictive lead scoring uses multiple data types including website interactions, email engagement, social media activity, purchase history, content consumption, demographic information, and firmographic indicators (company characteristics and individual metrics).
How does AI contribute to lead generation?
AI analyses patterns across multiple touchpoints to transform raw customer data into actionable insights. It evaluates leads through behavioural signals, engagement metrics, historical data, and company attributes, automatically ranking leads based on conversion likelihood.
What technologies are needed to implement predictive lead strategies?
Implementation requires a three-layer technology stack: foundation layer (data collection tools and CRM systems), analytics layer (machine learning algorithms and predictive analytics software), and execution layer (lead scoring models and engagement tracking tools).
How can success be measured in predictive lead generation?
Success is measured through specific metrics including lead quality score, conversion rates, engagement levels, and lead velocity. ROI assessment methods include attribution modelling and customer lifetime value calculations to evaluate financial returns against investment costs.
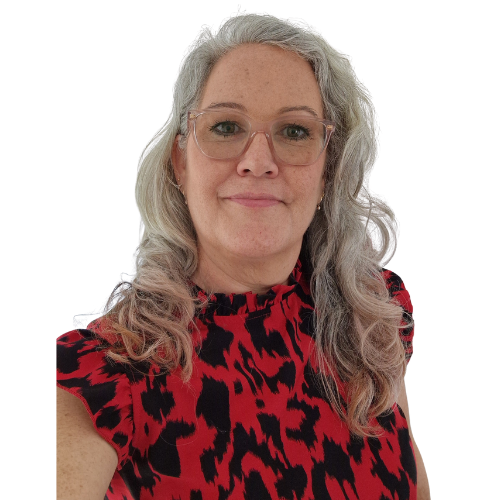